If you know me, you’ll know I’m not a fan of making tech predictions. It’s just not possible to consider the complexities of the world and the bucket of other unknowns. We also tend to be far more confident in our predictions. Humans, right? However, looking at some current trends leads to some pretty probable predictions. Besides, our marketing department loves predictions, so here is a Hunter S. Thompson style tagline of written under duress.
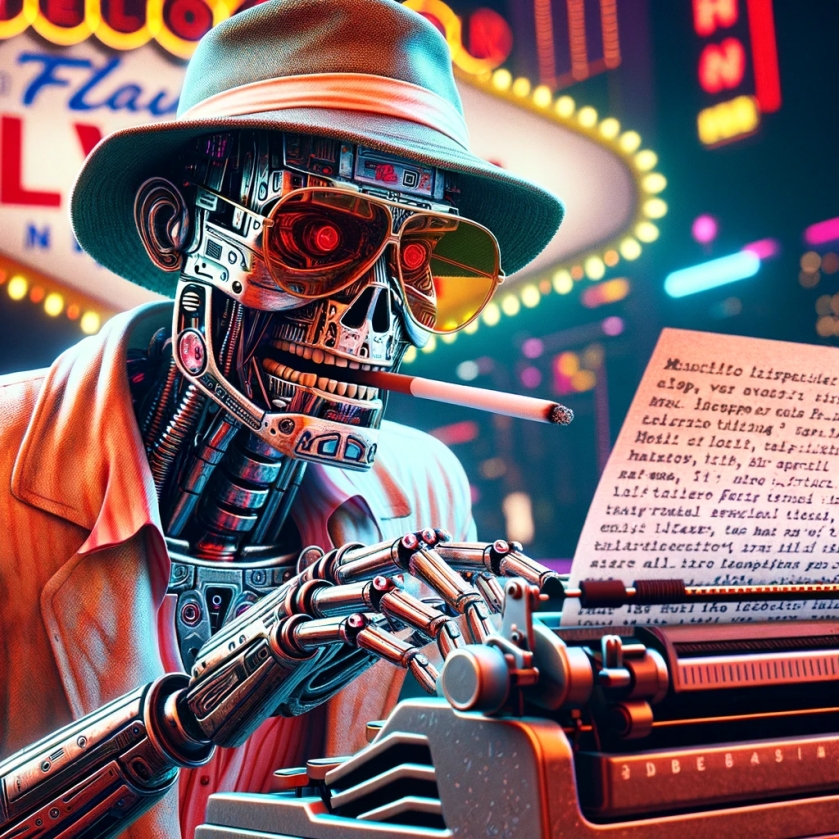
I want to take a different approach with these predictions than the typical hype-laden AI predictions (guesses) you typically see. I’ve added some context and food for thought with each prediction. I hope this is more enlightening than dropping a few bullet points.
Here is what I believe is in store for 2024.
Everyone at an Organization Will Be Encouraged to be a Developer
If you thought ChatGPT was causing your organization problems, it will get worse. In June, I started touching on this topic with my More than ChatGPT: Privacy and Confidentiality in the Age of LLMs post. It’s relatively easy for anyone to copy and paste some Python code and send data to an API. This post was before the announcement of GPTs, Microsoft’s Copilot Studio, and maybe whatever Amazon’s Q is supposed to be. These provide a better interface with more bubble wrapping. There’s no doubt more of these tools are on the horizon from other providers, and the complete reduction of friction is the goal.
The value proposition of these tools is allowing non-developers to use natural language to program new applications and deploy them for themselves or others. I like the spirit of empowering everyone at the company to create tools and solve problems, but there’s a reason we don’t let everyone at the company build and ship code. Non-developers aren’t accustomed to building and deploying software, much less knowing about issues with data and evaluating the output of software built. Even if these applications aren’t deployed outside of an organization (many may not be), they could expose data to compromise, lead to bad business decisions, and possibly put the organization in violation of regulatory compliance. The fact that they may be insecure is almost beside the point; the real problem is that security teams and the organization as a whole won’t know about them in the first place.
Many organizations continue to struggle with their more traditional development security challenges; now, many will have to worry about a new landscape of applications distributed across an organization. Most organization’s processes and approaches are entirely inadequate for what’s on the horizon. Much of this functionality is billed more as Excel on steroids vs. a development team pushing new software, but it’s still early. The time to prepare is now.
We’ve reached a point where just asking questions turns into code execution. Exciting.
Organizations Will Continue to Struggle With Generative AI Use Cases
The most significant hurdle to Generative AI adoption is the lack of appropriate business use cases. This is according to a November 2023 O’Reilly Radar Report titled Generative AI in the Enterprise. In addition, once you’ve identified a use case, operationalizing and getting it into production is another challenge. Use cases rarely operationalize as easily as the tutorials make it seem, and unexpected pitfalls tank the project.
Solutions often work in small tests, and the real problems don’t present themselves until they get launched into production and are confronted with the complexities of the real world. Before the generative AI craze, it was known that most AI experiments don’t make it into production, but for some reason, people treat generative AI as the exception.
The biggest companies in the world are struggling to operationalize these technologies in their environments, so we should expect this trend to continue to be a challenge into 2024.
Realization that Deep AI Integration is Bad for Both Security and Privacy
Imagine a world where you never know why any files are being accessed, you never know why your data is being sent anywhere, and you never know why code is changing and executing. You haven’t entered the Security Twilight Zone. You’ve entered our very near future. There’s a relentless push by vendors to “AI” everything and drive integration deeper into systems. What we tend to forget is that these technologies are experimental. We haven’t even found all of the issues with them yet and don’t have fixes for all the issues we’ve found, but every day, production pushes them deeper into systems.
Zooming out, there is a change in attack surface as systems that can be manipulated are integrated into previously robust applications. Allowing attackers to exert far greater control over these applications and manipulate them in unexpected ways.
Beyond security, there is a very real danger to privacy. Deep learning approaches are data-hungry, and there’s a temptation to use what you have access to. LLMs are typically worse for privacy because, most often, you need to be able to see the plaintext request and response to evaluate the quality of the generation. This evaluation, in many cases, is done by a human. Even when privacy protections exist in one product, it may not be the case in other product offerings from the company. So, it’s essential to keep an eye on the scope of these in your usage agreements and terms of service.
Impacts on security and privacy remain situational and depend on the use case. But the deeper the integration into products almost assures an elevated impact by the very nature of the depth of the integration. A whole lot of compromises will happen, and data will be lost and many will claim they never saw it coming. 🤷♂️
This prediction may be stretching it for 2024. We are still deep in the hype.
GPT-5 Won’t Be Much Better than GPT-4
When GPT-5 lands, it won’t be much better than GPT-4 and far from majorly better. With all of the hype and speculation around GPT-5 having near-AGI capabilities, the reality will surely be a letdown to the AI hype machine. We’ve reached a limit with the current LLM approaches, and bigger only buys you so much. So, without some new innovation, we are stuck relatively where we are. It’s possible that tweaks and additional modalities may make GPT-5 more useful for certain tasks, but not in a generalizable way.
I saw this image being passed around if you are looking for a visual aid.
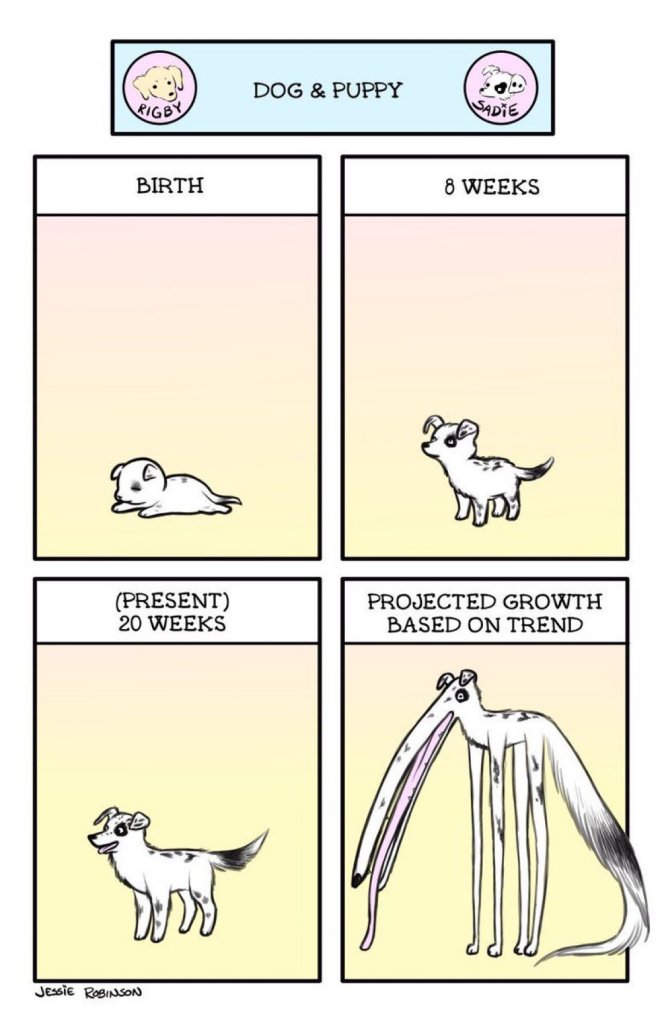
You can get a glimpse of this by looking at the current landscape of the various LLMs that have been released. It seems everyone is releasing an LLM, and none of them are substantially better than any other one. Sure, some may perform better at certain tasks, have additional modalities, or have been trained and fine-tuned differently, but they are all relatively the same.
Sustainability and Environmental Factors Enter the Conversation
The dirty little secret of Generative AI is the environmental costs, and it’s getting almost no attention. People railed against the negative environmental impacts of Proof of Work cryptocurrencies (and still are), but the same people are now silent on the environmental impacts of AI. Some of this is partly due to the fact that using these tools abstracts the user from the real costs of their transactions.
The following is from an AP news article covering the topic.
Ren’s team estimates ChatGPT gulps up 500 milliliters of water (close to what’s in a 16-ounce water bottle) every time you ask it a series of between 5 to 50 prompts or questions. The range varies depending on where its servers are located and the season. The estimate includes indirect water usage that the companies don’t measure — such as to cool power plants that supply the data centers with electricity.
This usage can also be particularly impactful when it happens during a drought.
Another new paper, Power Hungry Processing ⚡️Watts ⚡️ Driving the Cost of AI Deployment, also dives into this issue. This paper confirms a couple of assumptions that you may already have.
- Generative tasks are more energy and carbon-intensive than discriminative tasks.
- Tasks involving images are more energy and carbon investing than those that generative text alone.
- Training remains orders of magnitude more energy and carbon-intensive than inference
- Using multi-purpose models for discriminative tasks is more energy-intestine compared to task-specific models for these same tasks
It’s certainly something to consider before participating in the next AI-generated viral meme trend.
There are other sustainability factors at play as well. As long as there is little transparency and organizations rely on subsidies from big tech companies, the true cost of running these models isn’t known. It’s important for the sustainability of the service to know if you are paying $10 a month for a service that costs the company $30 a month to offer you. This is something Clem Delangue CEO of Hugging Face, calls “cloud money laundering.” This has an impact on organizations looking to deploy Generative AI solutions because the cost to deploy and maintain a service could skyrocket, making it less attractive or infeasible.
In 2024, this topic will start to be part of the public conversation, potentially creating a more precise understanding and additional research about the actual cost of the technology. I don’t expect any significant changes to happen in 2024, but having a better understanding can lead to proposed plans to help offset the impact.
The Big Innovation is Coming… Next Time.
If there is one prediction that I can make with 100% confidence, it’s that the goalposts will move. First was the access to the API; then, it was GPT-4; then, it was multi-modality; and now, it’s GPT-5, which will completely transform the world and be more impactful than the printing press.
It’s useful to remember that technology doesn’t have to be earth-shattering to be useful. We don’t need AGI to solve problems. I mentioned recently that I find my car’s driver assistance and safety features incredibly helpful, despite not having a self-driving car. I think we should be looking at AI technology the same way.
Many people and organizations are doing cool things with the technology today. It doesn’t have to be the new printing press to make an impact. So, stop hyping and start using.
Conclusion
Due to the factors outlined in this post and a variety of other factors, in 2024, we should see the hype around generative AI start to cool as certain realities set in and investors start asking tougher questions. This doesn’t mean AI is dead, far from it. Technologies under the AI umbrella are already part of our daily lives, and there will be continued advancements leading to solved problems.
Ultimately, we should prepare to be surprised. So many people are working in the area there are bound to be surprises. It’s time to start thinking critically about how we look at and deploy AI technology in our environments to ensure the right steps are taken to protect our assets. So, here’s to 2024.